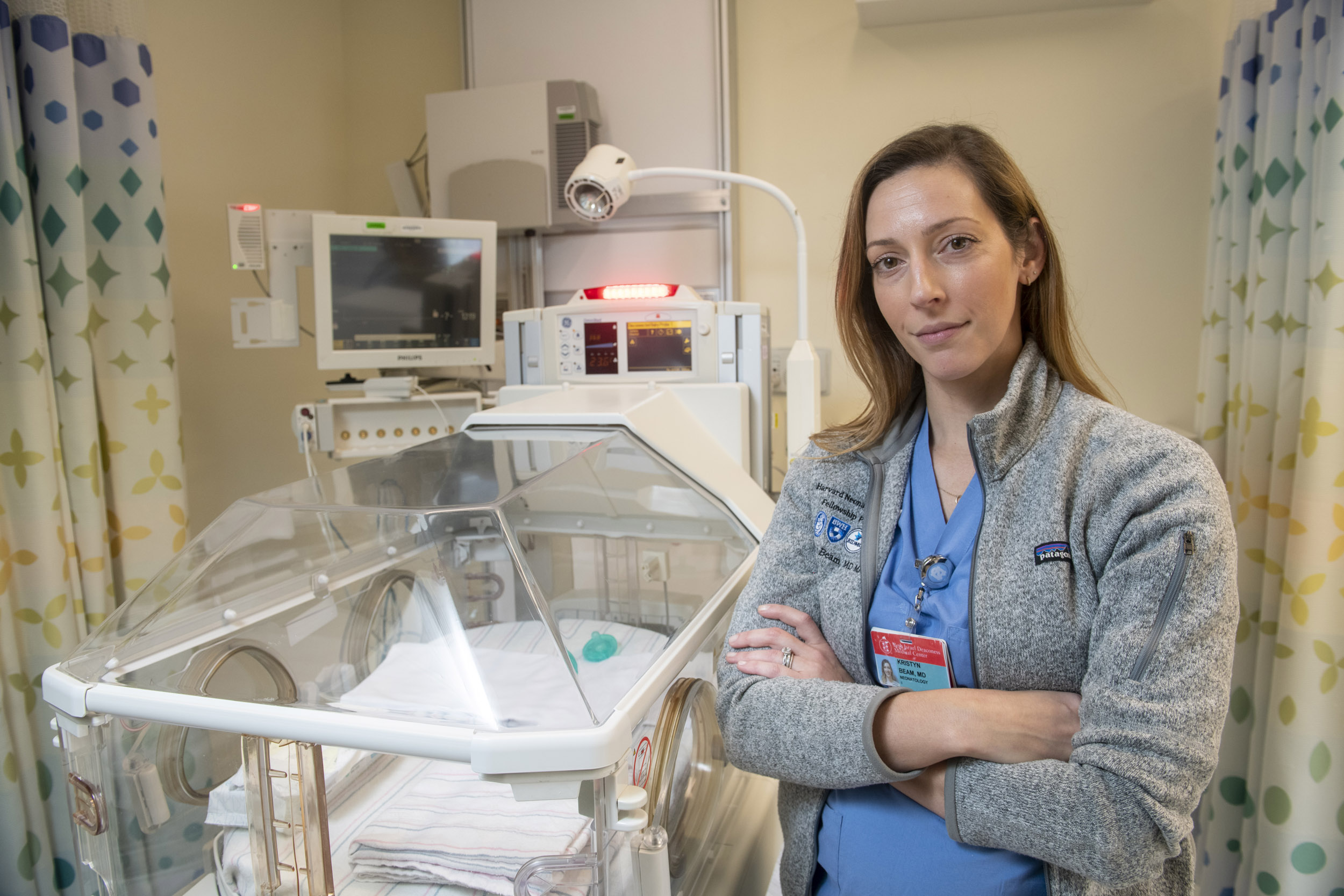
“It is really important to figure out how to bring that into the clinical world and to bring it in safely …,” said Kristyn Beam, who graded the ChatGPT’s responses.
Photos by Jon Chase/Harvard Staff Photographer
These doctors aren’t sweating AI — yet
Board exam for pediatric specialty stumps ChatGPT, at least in some areas
The ease with which ChatGPT can produce coherent content and convincing answers has raised fears that it will enable cheating on University campuses and replace workers in fields ranging from journalism to medicine.
A group of pediatric specialists, however, aren’t sweating just yet after their first pass at testing ChatGPT on the knowledge required to do their jobs.
Research conducted earlier this year pitted the 3.5 version of ChatGPT — a type of artificial intelligence called a “large language model” — against the neonatal-perinatal board exam required for practicing pediatricians specializing in the period just before and after birth. The AI got 46 percent correct.
The study, published in July in the journal JAMA Pediatrics, tested the large language model against a board practice test. It did best in questions of basic recall and clinical reasoning, and worse on more complex multi-logic questions. It performed poorest, 37.5 percent, in gastroenterology, and best, 78.5 percent, in ethics.
The study’s senior author, Andrew Beam, assistant professor of biomedical informatics at Harvard Medical School and of epidemiology at the Harvard T.H. Chan School of Public Health, said he knew that ChatGPT had successfully passed some general professional examinations, including the U.S. Medical Licensing Exam, required for medical students to become doctors. But he wondered how it would fare against more specialized board exams, taken by physicians who’ve devoted additional years of study and clinical work to master more narrowly focused specialties. Luckily, he didn’t have far to look.
Beam’s wife, Kristyn, an instructor in pediatrics at Harvard Medical School and a neonatologist at Beth Israel Deaconess Medical Center, agreed to participate by grading the AI’s answers along with HMS colleague Dara Brodsky, author of an influential neonatal textbook, and her co-author Camilia Martin, chief of newborn medicine at Weill Cornell Medicine and New York Presbyterian-Komansky Children’s Hospital.
The speed of development of these latest large language models have impressed Andrew Beam, who advocated pitting AI against the U.S. Medical Licensing Exam back in 2017 at a technology conference but found his own models couldn’t do better than 40 percent. Then things started moving quickly.
“There was this moment last year when, all of a sudden, five or six different models were all getting scores of 80 percent of higher,” he said. “The pace in this field is just crazy. The original ChatGPT isn’t even a year old — even I tend to forget that. But we’re very, very early in this and people are still trying to figure things out.”
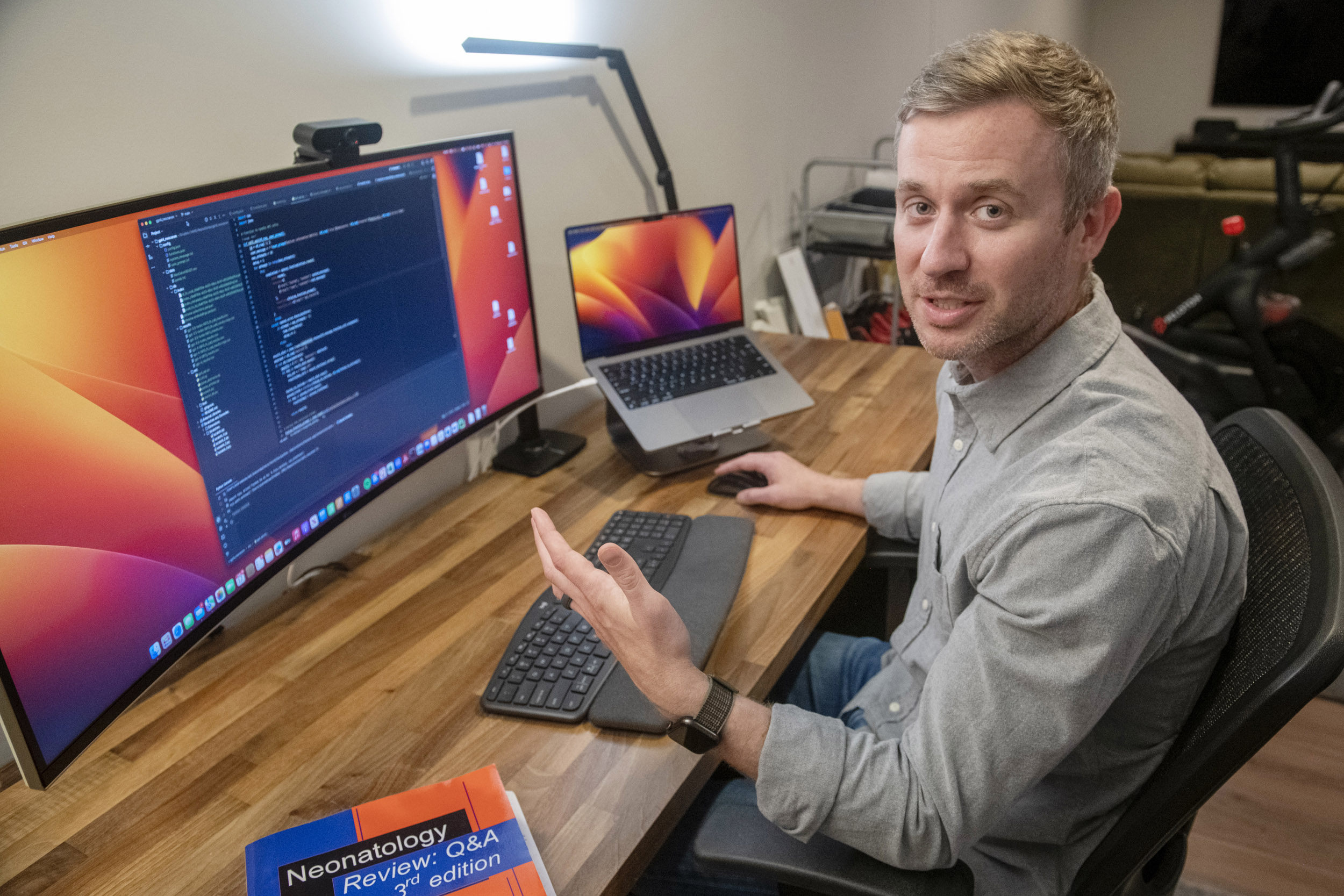
Kristyn Beam, the paper’s first author, also has been impressed with the AI’s capabilities — though she admits rooting against it on the test.
“I wanted it not to do well, so from that perspective I was happy,” she said. “It’s a little bit of an existential thing, where you’ve trained for decades to be able to do all these things, then a computer can just come and all of a sudden do it.”
She realizes, however, that not only will newer versions of the model perform better — they’re now testing the next iteration, GPT4, against the same test and against the anesthesiology board exam — but that once humans figure out what it can and can’t do, it will be a potentially powerful tool in doctors’ offices and hospital clinics.
“I think if you move past that initial resistance and say, ‘This is coming, how can this actually help me do my job better?’ then you can move past the feeling of ‘What have these past several decades been for? What did I do all this hard work for?’” she said. “It is really important to figure out how to bring that into the clinical world and to bring it in safely, so that we’re not affecting patients in a bad way but using every tool available to us to deliver the best care we can.”
Part of that process will depend on understanding what these large language models are and why they do what they do, said Andrew Beam, who is the founding editor of a new journal, NEJM AI, focusing on AI in medicine.
These models are fundamentally prediction machines, he said, and are extraordinarily sensitive to prompts while insensitive to things a human respondent might think important, like what the user actually wants or even whether the answer is right.
For more technical requests, in fact, wrong answers may be common simply because most of the humans answering the question got it wrong. A workaround, he said, is in the prompt, asking the model to answer as if it is an expert or the smartest person in the world.
“Imagine that it’s read 1,000 questions that are very similar, and imagine that it’s a very difficult question and it’s reading it on posts and nine times out of 10, the text that it sees coming next is wrong. The average completion it actually sees is wrong,” Andrew Beam said. “But it’s able to kind of triangulate and know that the really smart people actually get this right. … Often the default completion is the correct one, but in some instances, it’s not. You have to kind of trick it into giving you the correct one.”
Another issue is what are called “hallucinations” where, if the answer isn’t in its data set, the large language model can make things up, including sources formatted to look quite convincing, while entirely imaginary.
It’s important to be aware of these limitations, but Andrew Beam said he doesn’t think they’ll be problems for long. None of them are problems of fundamental theory, he said, and workarounds are already being devised. Creating prompts that result in correct answers has been recognized as important enough that “prompt engineering” has become a new job description.
“I think of it almost like incantations where you have to say the right mystical phrase to the AI to get it to do the thing you want it to do,” Andrew Beam said. “A lot of people don’t realize that it will just happily make things up that sound completely, completely realistic.”
A corollary to all this, Beam said, is that it is important to know which version of a particular large language model you’re using. For example, ChatCPT 3.5, released late last year, is still freely available on the company website even though another version, GPT4, is more accurate. That version is available on a subscription basis. Most users will likely be attracted to the free tool and should keep in mind its limitations, he said.
“AI has been the thing that I’ve been interested in for 15 or 20 years and it has always been something that will happen, not something that is happening,” Andrew Beam said. “I definitely feel like something is happening now. This feels qualitatively different.”