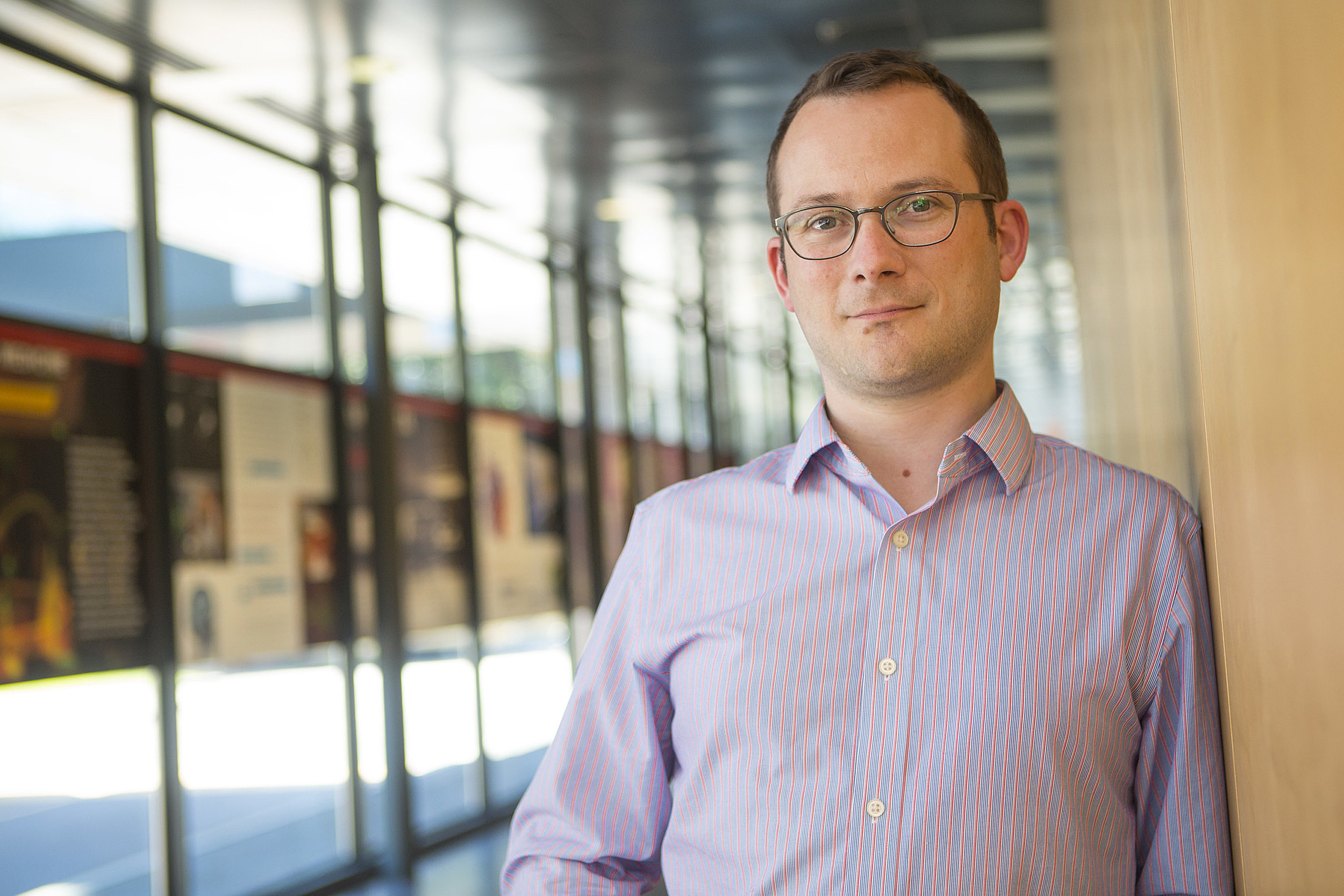
David Parkes, the George F. Colony Professor of Computer Science, talks about the emerging field of machine behavior.
Courtesy of SEAS Communications
The science of the artificial
Researchers propose a new field of study to explore how intelligent machines behave as independent agents
In 1969, artificial-intelligence pioneer and Nobel laureate Herbert Simon proposed a new science, one that approached the study of artificial objects just as one would study natural objects.
“Natural science is knowledge about natural objects and phenomena,” Simon wrote. “We ask whether there cannot also be ‘artificial’ science — knowledge about artificial objects and phenomena.”
Now, 50 years later, a team of researchers from Harvard, MIT, Stanford, the University of California, San Diego, Google, Facebook, Microsoft, and other institutions is renewing that call. In a recent paper published in the journal Nature, the researchers proposed a new, interdisciplinary field — machine behavior — that would study artificial intelligence through the lens of biology, economics, psychology, and other behavioral and social sciences.
Intelligent machines, the researchers argue, can no longer be viewed solely as the products of engineering and computer science; rather, they should be seen as a new class of actors with their own behaviors and ecology.
The Harvard John A. Paulson School of Engineering and Applied Sciences (SEAS) spoke with David Parkes, the George F. Colony Professor of Computer Science and co-author of the paper, about this emerging field and what the future has in store for intelligent machines.
Q&A
David Parkes
SEAS: For so long, the study of artificial intelligence and intelligent machines has been confined to the realm of computer science, and the researchers who built the machines were the same ones who studied their behavior. Why is it important to expand the scope of study to include new fields, including behavioral and social sciences?
PARKES: First, a separation between the designers and builders of intelligent machines and those who study how they are used (or not) can bring an independent viewpoint in developing and testing the right sets of hypotheses about the performance of these technologies. There are pragmatic reasons too, in that the study of intelligent machines becomes a behavioral science, requiring quite different kinds of expertise. Another point is that systems developed in the narrow confines of a lab may behave very differently “in the wild,” when behavior becomes a product of the way in which they are used, including the many ways that are different from what their designers had intended. Microsoft’s Tay bot [which began posting offensive tweets after trolls “taught” her hate speech] is one unfortunate but not-so-unique example.
SEAS: How might the fields of machine behavior and computer science grow together and inform each other moving forward?
PARKES: As computer science has come to have such impact, the field has come to embrace what economists might refer to as “positive analysis,” which is to say analysis that is based on the empirical and experimental studies of deployed, computational systems — the structure of the World Wide Web, the propagation of information on social networks, or the way in which interactive tutoring systems are used, to give just three examples. Intelligent machines are a new kind of artifact that we need to study and understand, and we’ll need to do this in an interdisciplinary way that includes computer scientists working collaboratively with social scientists, humanists, ethicists, legal scholars, to name just a few. More broadly, the study of machine behavior will be impacted by advances in data science, in working at scale with vast amounts of different kinds of data, and in leveraging methods of probabilistic machine learning and statistics to tease out cause and effect.
SEAS: Your work focuses on the intersection of AI and economics. What questions of machine behavior are you most interested in answering?
PARKES: I am interested in a research program that studies machine behavior within the algorithmic economy, including pricing algorithms, recommender algorithms, and reputation systems, as well as in the context of blockchains. We can already see a trajectory toward the automation of many of the core constituents of what makes up an economic system, and the machine behavior lens is a good one because behavior is emergent, meaning it’s based not only on individual interactions but also on societal and economic forces. I think recommender systems such as those employed by Amazon are especially interesting and important to study because that’s where we’ll see thorny questions arise around behavioral economics, algorithmic marketing, and ethics … For example, is it okay for an intelligent recommender to leverage “choice set effects” to drive up revenue?
“There is a need to move forward deliberatively … while at the same time with the recognition that people and machines will continue to become bound together in new and unexpected ways.”
SEAS: What are choice set effects?
PARKES: I show you a cheap, moderate-cost, and expensive coffee machine and you pick the moderately priced one. But, if I show you a moderate, expensive, and uber-luxury machine, you pick the …?
SEAS: Expensive one.
You brought up private companies such as Amazon and Microsoft. Proprietary and black-box algorithms must pose a challenge to understanding machine behavior. How can we understand why a machine behaves the way it does when we don’t know what the algorithm is or how it makes decisions?
PARKES: Funnily enough, the algorithms need not themselves be very complicated. The algorithms for training a deep-learning system, which describe the architecture of a model and the way in which a model will be trained, can typically be expressed in just tens of lines of code (albeit code that then builds on top of other, lower-level code). It is the trained models that are complex and somewhat inscrutable, often considered to be a “black box.” But it is not hopeless, and there are many sensible research directions — for example, requiring simpler models, insisting on a post hoc explanation of the behavior of complex models, and using visualization and sensitivity analyses to try to understand the way these models work and test theories about behavior.
SEAS: Artificial intelligence already plays such a large role in our lives. What is the importance of establishing this new field of research now? Are you afraid it’s being started too late, when so much of the foundation of AI has already been laid?
PARKES: Well, it’s never too late, and we’re only at the beginning of the wave of change that will come from the development of intelligent machines. There is a need to move forward deliberatively, with appropriate measures of curiosity, creativity, and responsibility, while at the same time with the recognition that people and machines will continue to become bound together in new and unexpected ways. What’s important is the recognition of the need for scientific study, and this review article brings together threads in this emerging, interdisciplinary field of machine behavior.